TextFooler fools BERT
TextFooler fools BERT
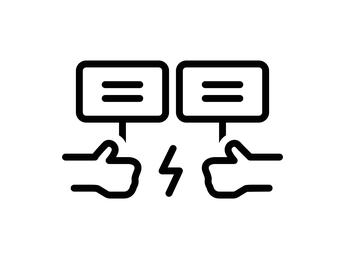
It was a humbling moment for the state-of-the-art NLP models when an adversarial test compromised the output significantly. Yes, this included BERT as well, where its classification task prediction accuracy in a set of text analytics tasks reduced by 5 to 7 times!
TextFooler is a baseline framework for synthetically creating adversarial samples, was created by a team of researchers in the US, Singapore and Hong Kong. Consider this: among other datasets, the team used Yelp reviews dataset for sentiment classification of sentences. On BERT, the original accuracy was 97 – and the after-attack accuracy was an abysmal 6.6.
The system identifies the most important words in the target model and replaces them with semantically similar and grammatically correct words – and it keeps doing that till the prediction is altered. An example quoted in the VentureBeat article has “impossibly contrived” replaced by “impossibly engineered”. Small, subtle changes like these apparently unravel the models’ efficacy.
Deep learning model improvements through adversarial training will be a key focus area to build truly robust NLP models. Read about the TextFooler details here.
Image Source: Shutterstock